Currently, financial institutions are becoming increasingly dependent on the power of Machine Learning (ML) to improve their operations, optimize decision making and provide more personalized services to their customers. That is why we at ITSense have prepared this article where we will explore several use cases of Machine Learning in the financial industry and the tangible benefits it brings to financial institutions .
Fraud Detection
One of the most prominent use cases for Machine Learning in the financial industry is fraud detection. ML algorithms can analyze large volumes of transactional data and identify suspicious patterns that could indicate fraudulent activity. For example, a bank can use ML models to detect unusual transactions, such as purchases outside the usual spending pattern or suspicious activity in bank accounts.
Case Study: PayPal uses Machine Learning algorithms to detect fraudulent transactions in real time. Its system analyzes thousands of variables to assess the probability of fraud in each transaction and automatically takes preventive measures.
2. Credit Risk Assessment
Another common use case for Machine Learning in the financial industry is credit risk assessment. ML algorithms can analyze historical customer data, including payment habits, credit history and financial behavior, to predict the risk of defaulting on a loan or credit.
Case Study: LendingClub, a peer-to-peer lending platform, uses Machine Learning models to assess the credit risk of loan applicants. Its system analyzes data from thousands of applicants to predict the probability that a borrower will not repay the loan .
Did you know that at ITSense we have an Open Data tool that will facilitate your risk assessments? Learn more.
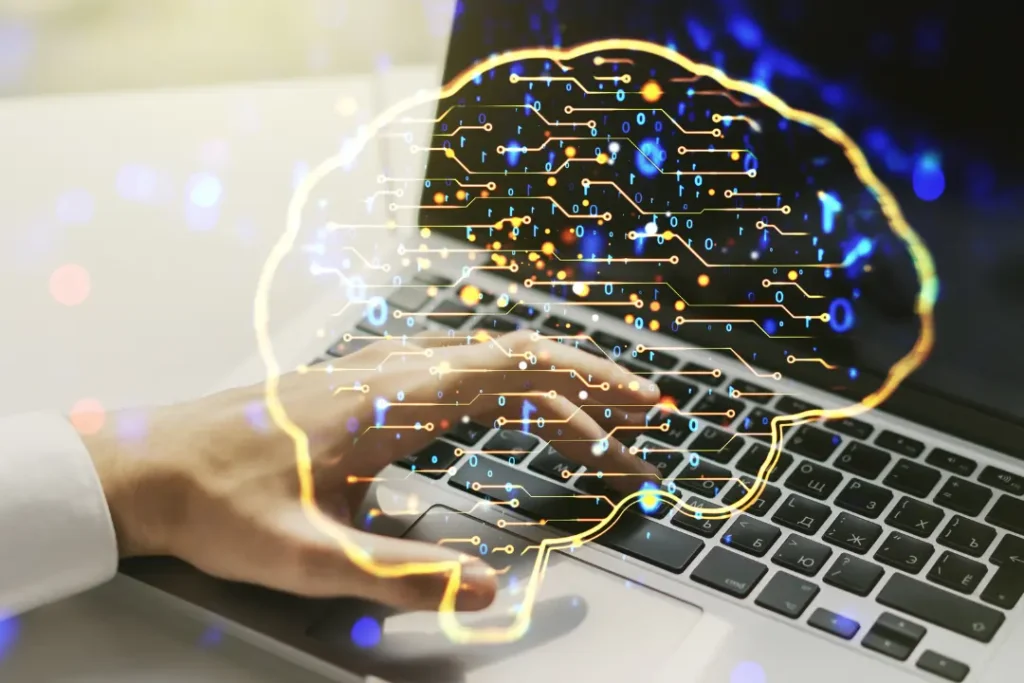
3. Personalization of Financial Services
Machine Learning is also used to customize financial services according to individual customer needs and preferences. ML algorithms can analyze customer behavior, past transactions and other variables to provide personalized recommendations, products and services tailored to each user .
Case in point: Bank of America's mobile banking app uses Machine Learning to provide customers with personalized recommendations, such as savings tips, product offers and spending notifications.
Benefits for Financial Institutions
Improved operational efficiency: Machine Learning automates repetitive tasks and optimizes internal processes, helping financial institutions improve operational efficiency and reduce costs.
More informed decision making: ML algorithms analyze real-time data and generate valuable insights that help financial institutions make more informed and strategic decisions.
Improved customer experience: ML-based personalization of financial services enables financial institutions to deliver a more relevant and satisfying experience to their customers, which increases customer loyalty and retention.
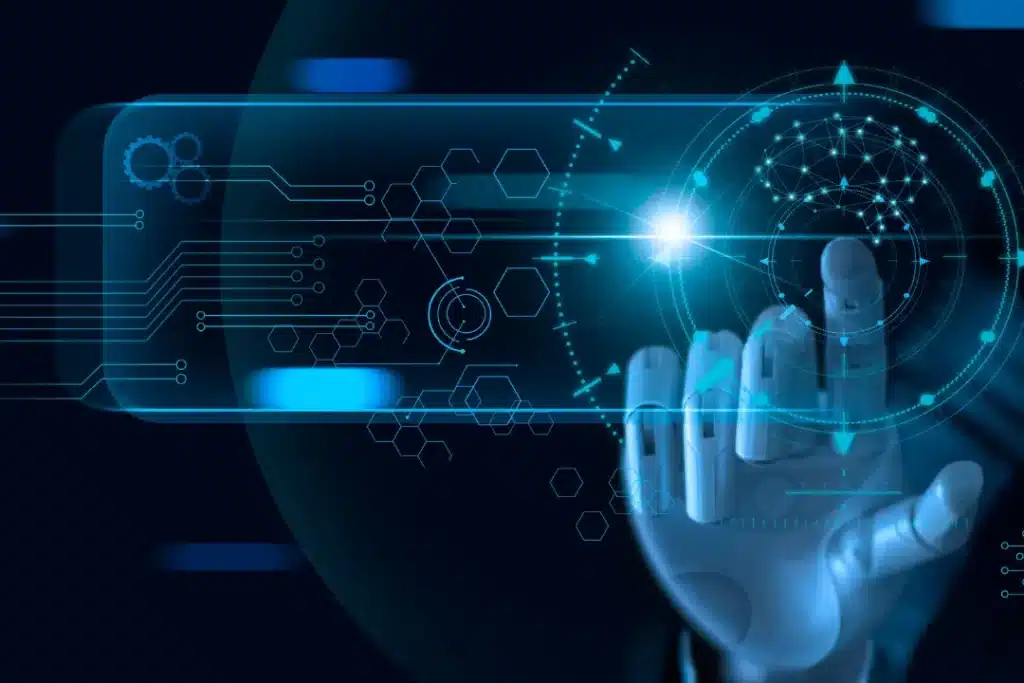
In short, Machine Learning is transforming the financial industry by providing innovative solutions to complex problems. By adopting these advanced technologies, financial institutions can improve efficiency, reduce risk and offer more personalized services to their customers, enabling them to remain competitive in an ever-changing marketplace .
If you want to implement or improve your processes with Machine Learning, contact us and we will generate the best plan for you .